Effective operationalization of AI governance requires more than qualitative frameworks—it demands innovative, dynamic metrics that provide actionable insights. Drawing from recent research on strategic measurement systems, how can organizations develop AI governance KPIs that track compliance and drive strategic value and competitive differentiation?
Findings from the MIT SMR - BCG Artificial Intelligence and Business Strategy Global Executive Study and Research Project reiterate the need for an evolution in the KPIs businesses use to measure advanced AI technologies, their implementation, and, in particular, governance.
As the authors share, "Smart KPIs can do more than just unearth sources of value and differentiation that would otherwise go undetected or underexploited. They can also prevent the undesirable outcomes that can result from a failure to regularly reexamine assumptions underlying legacy KPIs. The 2008 global economic crisis, for example, was triggered in part by banks’ dependence on a then widely used metric, value at risk, which measures potential portfolio losses in normal market conditions at a single point in time. Financial institutions did not adjust this measure as riskier subprime mortgages and credit default swaps became a larger part of their portfolios. Guided by a metric that severely underestimated potential losses — in some cases, by orders of magnitude — many financial institutions went bankrupt or suffered significant losses."
Developing Clear KPIs for AI Governance
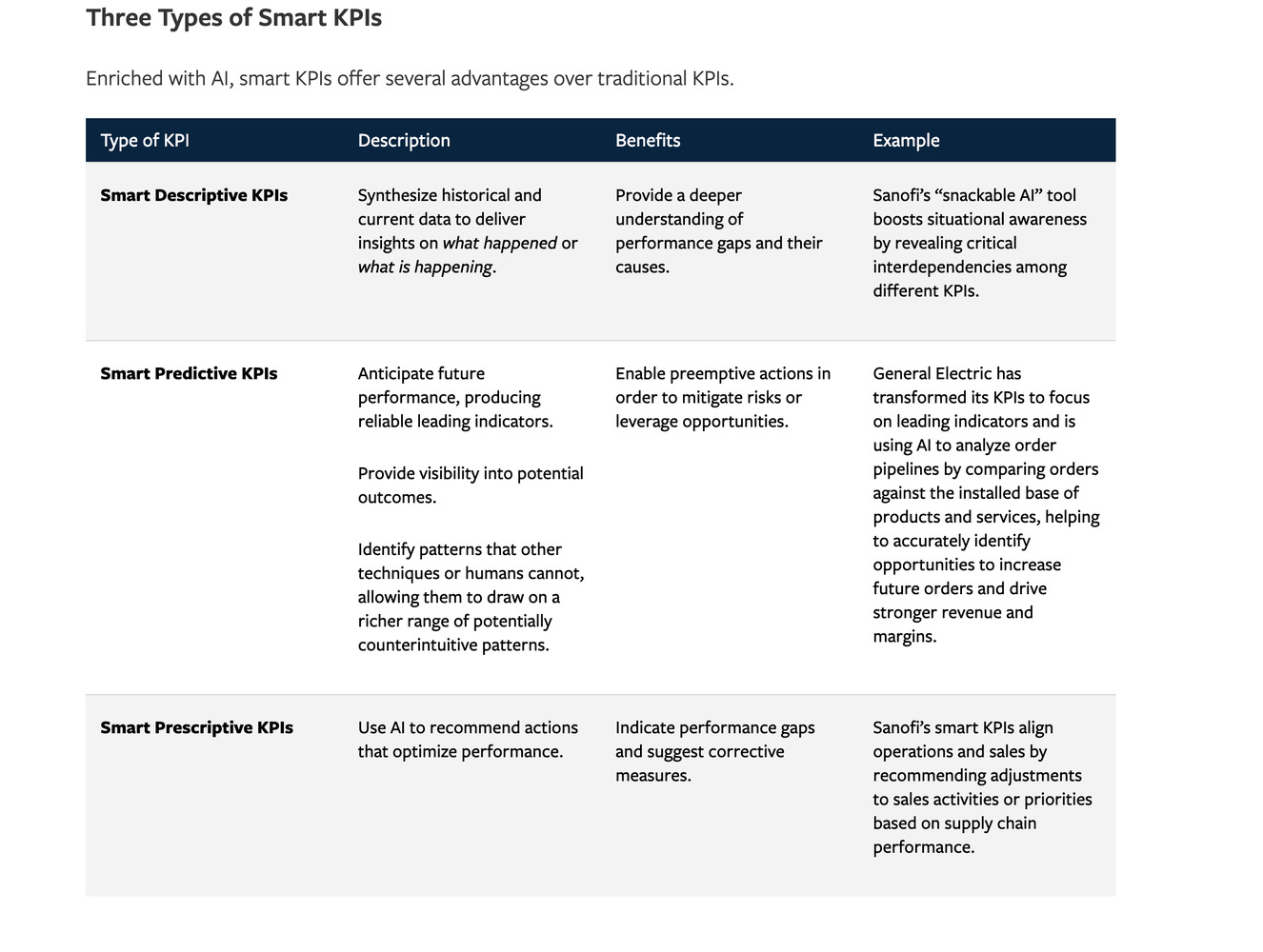
The traditional approach to governance measurement often fails to deliver the intelligence and adaptability required in today's AI landscape. Following the research from MIT Sloan Management Review and BCG, we propose shifting governance KPIs from static benchmarks to dynamic predictors that anticipate risks, identify opportunities, and align with strategic objectives.
Effective operationalization of AI governance requires clear metrics to track progress and identify areas for improvement. While governance might seem inherently qualitative, leading organizations have developed sophisticated Key Performance Indicators (KPIs) that provide quantitative insights into governance effectiveness.
The foundation of governance measurement begins with inventory coverage—tracking what percentage of an organization's AI systems are known and governed. This baseline metric reveals whether the governance framework is comprehensive or if "shadow AI" exists outside formal oversight. A related metric examines policy compliance rates, measuring how consistently AI projects follow required governance steps before deployment. Both metrics should trend toward 100% as governance matures, though achieving perfect compliance often requires sustained effort.
Data quality and lineage metrics form another important measurement category. Since responsible AI depends on good data, organizations track how completely the origin of data for each model is documented (data lineage completeness) and how well that data meets quality standards (accuracy, completeness, representativeness). Many governance programs set minimum thresholds for data quality scores that models must meet before approval.
Bias and fairness metrics have become particularly crucial in recent years. Organizations define specific thresholds for acceptable differences in model outcomes across demographic groups, such as requiring disparate impact ratios above 0.8 for protected attributes. They track the initial bias measurements during development and how these metrics trend over time in production. A well-governed AI system should maintain or improve fairness metrics throughout its lifecycle.
Model performance and stability represent both technical and governance concerns. While traditional accuracy metrics remain important, governance frameworks also monitor drift rates—how frequently models deviate from expected performance parameters—and the frequency of required updates due to drift. Some organizations even track "technical debt" in their AI models, quantifying how much rework or complexity accumulates over time and might require addressing.
Incident metrics provide the most direct insight into governance effectiveness. These include the number and severity of AI-related incidents, mean time to resolution, and trends in incident occurrence. As governance matures, the expectation is that severe incidents should decrease, though increased reporting of minor issues might initially suggest the opposite as awareness improves.
Accountability and training metrics track the human elements of governance. Organizations measure the percentage of relevant staff who have completed AI governance training, the frequency of governance council meetings, and the level of stakeholder participation. Some even track governance responsiveness—how quickly the governance body reviews and approves AI projects—seeking a balance between thorough oversight and operational efficiency.
Value and ROI metrics connect governance to business outcomes. These might include the number of AI projects successfully deployed versus those halted due to governance concerns or the financial value generated by AI systems compared to losses or fines from AI failures. Positive trends in these metrics indicate that governance enables valuable AI while preventing harmful applications.
A more integrated measurement approach can transform AI governance from a nebulous concept into a quantifiable discipline. By tracking these metrics in dashboards accessible to technical teams and executives, organizations create accountability for governance outcomes at all levels. Regular review sessions examine metric trends, identify impr